Optimizing data loading for text analytics
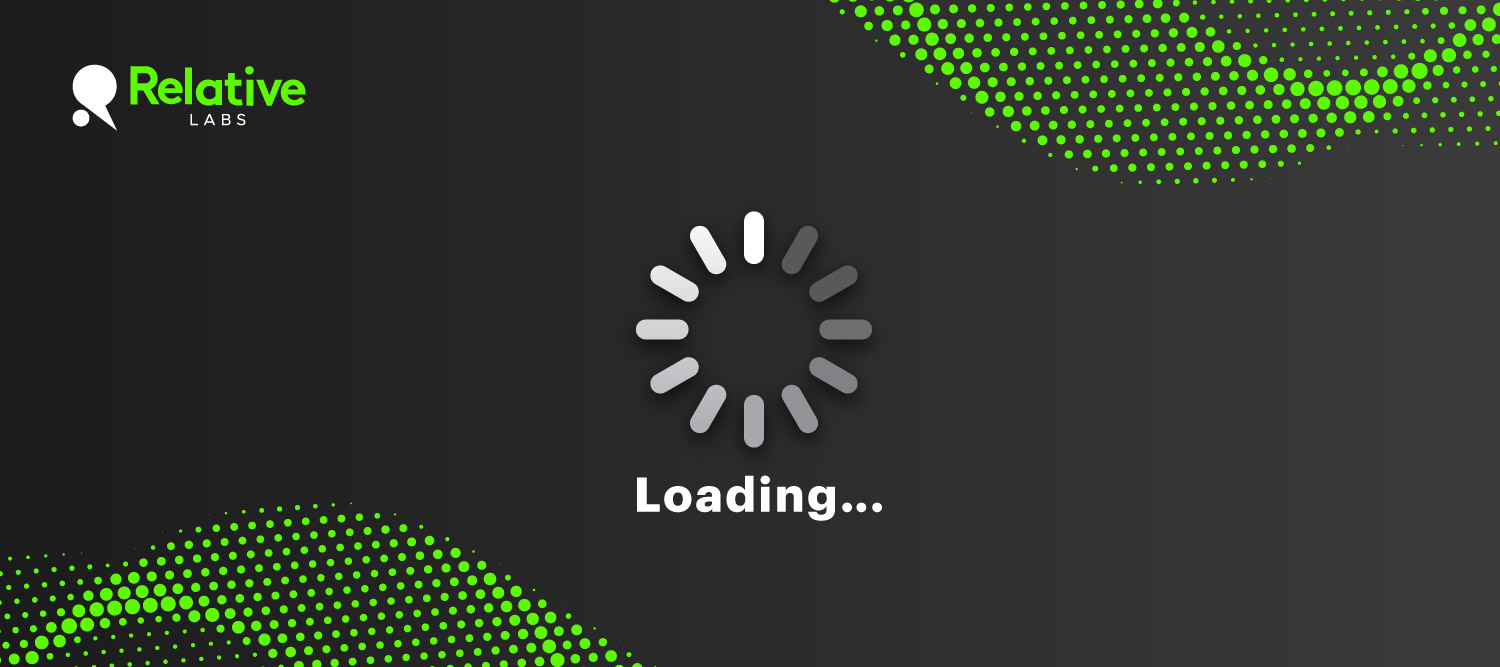
Many of our previous blog posts at Relative Labs have delved into cutting-edge techniques for maximizing the value of your text assets. However, all these strategies become futile if you encounter difficulties in accessing and loading your data into the desired platforms. It’s akin to owning a high-performance sports car without access to fuel!
At Relative Insight, we annually analyze thousands of our customers’ data files, encountering various file types and structures.
Through this experience, we have identified common challenges that our customers face during data processing. This article outlines these challenges and offers effective solutions.
No structure
Text data is typically seen as unstructured data, and uploading data as a .txt or .docx file to the Relative Insight platform is fine.
However, data with no structure at all can sometimes be an obstacle; typically encountered when working with transcribed data from call centers or interviews.
In these cases, it is often desirable to isolate the different speakers in the conversation. For example, you can analyze the operator and customer side or the interviewer and interviewee side of the conversation separately.
We have technical teams within Relative Insight who have vast experience and tools available to apply the desired structure to this type of data and we’re actively working on automated solutions to this problem.
Metadata consistency in data loading
Metadata plays a crucial role in the analysis process, especially when investigating specific data segments.
Typically metadata used within the Relative Insight platform includes: date/times, NPS segment, CSAT scores, location, etc. Consistency in metadata values is essential to ensure accurate exploration of different segments.
We see a common inconsistency in date formats, for example, switching between the US format of MM/DD/YYYY and the format used elsewhere in DD/MM/YYYY.
Our Smart Uploads feature detects the correct date format and provides a preview of your metadata, allowing you to choose the columns you want to include or exclude to guarantee consistency.
Text encoding
While you can upload unstructured .txt files, text encoding and character sets can pose challenges. Text encoding is a set of rules that allow computers to translate letters, numbers, and symbols into 0s and 1s, and character sets are like different languages within those rules.
Different operating systems and applications default to different encoding and character sets, some languages also require a specific character set and if the wrong combination is used to read the text files then problems occur.
At Relative Insight we automatically detect the correct encoding and character sets to use for our supported languages. For unsupported languages, you can consult your account manager for guidance.
Poorly structured CSV files during data upload
CSV files are widely used for tabular data transfer due to it being a generic format and not tied to any particular vendor.
CSV stands for comma-separated values and as the name suggests the plain text column values are delimited using commas.
Issues can arise when column values contain commas or use alternative delimiters like tabs in .tsv files. This relies on the .csv creator to correctly follow conventions, and not all of them do.
In these cases Excel and other spreadsheet applications can be your friend – these applications are generally very robust and will detect problems and guide you on the best way to correct them.
Data duplication
Some data sources can be particularly prevalent with duplicate or near-duplicate content, typically seen in social media content due to re-tweets or people sharing the same articles.
Often you will want to filter out this content to increase accuracy. During the upload process, the Relative Insight pipeline will attempt to detect exact or near duplicate content, this content can be subsequently filtered or included in your analysis.
Other types of undesirable content can also be excluded including spam and marketing material.
Automate your text analytics and visualizations with Relative Flow
Tips for best practice for data loading
In addition to these specific challenges, here are some general tips to streamline your data-loading process:
Inspect your data files
This can uncover some obvious problems, for example, does the file even contain data that can be analyzed?
Use Smart Uploads
Relative Insight’s Smart Uploads provides a visual display of your file’s contents. It also allows you to select which data should be included or excluded. This allows you to omit any unnecessary (and potentially problematic) data.
Data cleaning
Apply the data cleaning techniques offered by Relative Insight to remove duplicates, spam and marketing material.
Use the tools you already have
Using applications such as Excel can remedy a lot of common problems. For example, re-saving .csv files to .xlsx can fix structural problems.
Fix issues as early as possible
“Prevention is better than cure” so always try to plan and design your data collection process as soon as possible. Think about the source of your data to ensure it is high quality. Then use standard off-the-shelf packages to create your data, and use well-structured data formats to store your data.
Speak to your data controllers or engineers
If you have people responsible for managing and providing the data in your organization, speak to them to see if they can help. How the data is stored in its original source might not be the same as how it’s provided. They might be able to export the data in different formats or filter it in different ways.
Use Relative Flow
Relative Flow is an extension to the Relative Insight platform that automates common operations.
Relative Flow can be used to automate data uploads whilst performing necessary data manipulation to ensure the data is ready for analysis. Furthermore, Relative Flow can ingest data directly from its source eliminating the need to work with brittle export files. Speak to your account manager to learn more about Relative Flow.
Efficient data loading is the foundation for effective analysis. By overcoming these challenges and following these tips, you can ensure that your data is primed and ready for insightful analysis.
Stay tuned for updates on our ongoing efforts to enhance data-loading capabilities!
Leave a Comment