Demystifying text mining: a jargon-free guide
New tools and technologies bring with them a minefield of jargon that can feel near impossible to navigate – text mining is no exception!
Between text analysis, machine learning, NLP, sentiment analysis software and AI, you might find yourself with more questions than answers.
In this accessible, jargon-free guide to text mining, we’ll answer these key questions (and more) to help you navigate your new secret weapon for better business intelligence.
What is text mining?
It’s a lofty-sounding phrase with a surprisingly simple meaning:
Text mining is the act of analyzing large quantities of text data to uncover objective insights.
Despite this simple meaning, sometimes the process can seem complex.
Words can take on context-specific meanings that make them more challenging to analyze reliably than numbers.
Traditional BI tools like PowerBI and Tableau are not built with text data as their core focus, and the analytical outputs reflect this.
This has led organizations to analyze this data in a haphazard, human-driven way (or not at all). Because of this, text data is rarely utilized to its full potential.
NLP text mining combines linguistic expertise and machine learning-powered automation to deliver qualitative analysis at scale.
Like any kind of mining, AI text mining – also referred to as text analytics or text processing (just to keep you on your toes) – is about finding the inherent value hidden within the pile of text data.
Text mining vs. text classification
So is text mining the same as text classification?
Well, yes and no.
In its simplest form, text mining involves classifying text data to understand common themes.
This can be done on a granular word or phrase level, or on a broader topic or emotional level. You can report on how often particular features occur in a data set or look at the percentage of messages where a particular topic was discussed, as examples.
But the best text mining tools offer more than simple text classification.
These tools make it easy to segment and compare data sets based on associated attributes, like location, satisfaction rating, or other demographic or behavioral data points. They also provide a suite of visualizations to understand what audiences are saying or how things are changing at a glance.
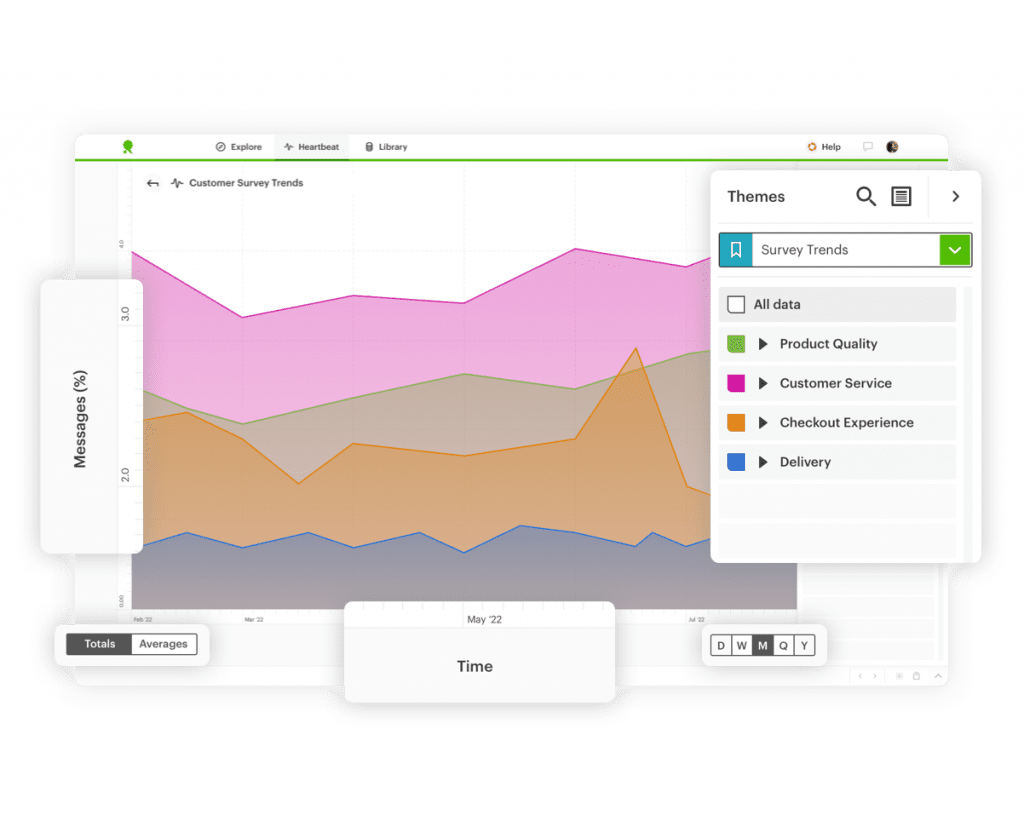
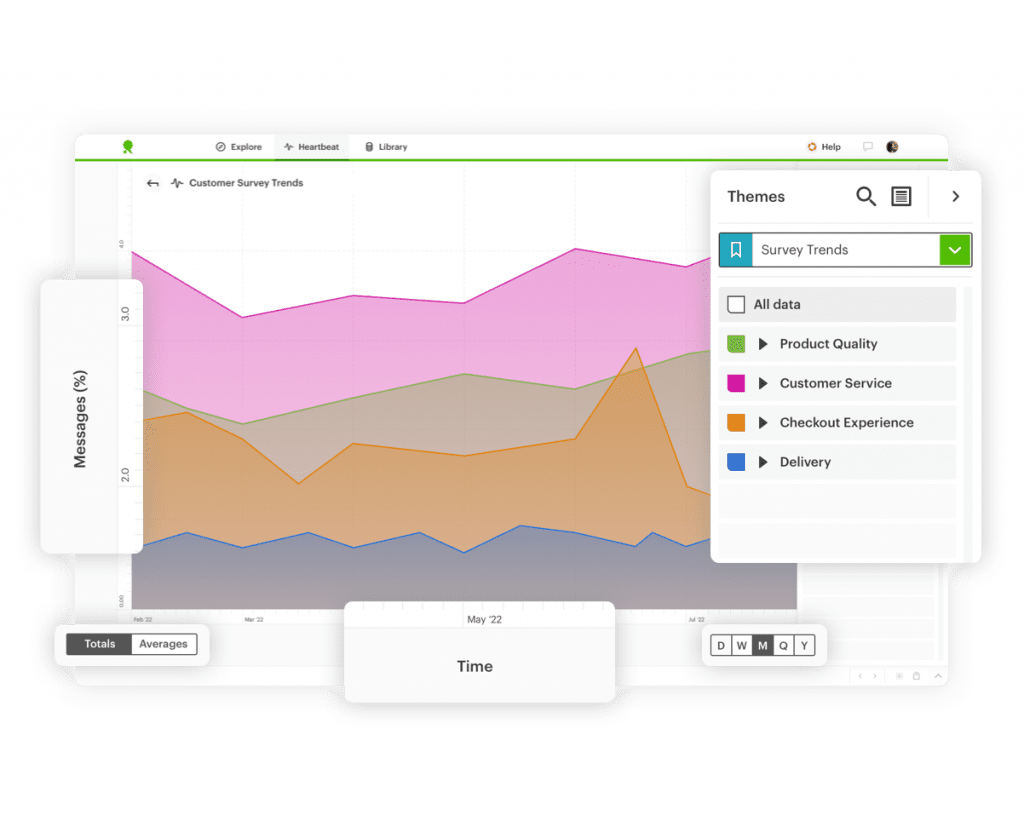
In other words, where text classification walks, AI text mining runs.
What is the relationship between text mining, NLP, and machine learning?
It all sounds very complicated, but when you break down the jargon it all becomes clearer.
Let’s return to our grounding definition of text mining: the act of analyzing large quantities of text data to uncover objective insights (AKA qualitative analysis at scale).
Machine learning and natural language processing (NLP) are two essential elements that help to power this analysis.
More specifically, NLP refers to the series of processes that computers undertake to understand and perform analysis on a body of text, while machine learning is used to improve the accuracy with which text mining algorithms can determine what a text is about.
This is an especially difficult task when you consider that words can mean very different things in different contexts. The simple word ‘type’, as an example, takes an alternative meaning in the phrases “he can type over 100 words per minute” and “their really not her type”.
Where text mining is the overall act of qualitative analysis at scale, NLP and machine learning are two key components of the engine that makes it happen.
Find out what insights you’ve been missing!
Are emotion and sentiment analysis part of text mining?
Sentiment analysis has become synonymous with social listening tools, where positive, negative, or neutral classifications often oversimplify how customers and target audiences feel about your brand. Knowing whether they like it, dislike it, or feel a bit ‘meh’, isn’t especially insightful.
But the best text mining solutions have the potential to deliver deeper emotional insights.


Insights that can help you understand the positive emotional connections you have with your consumers and build on them. And insights that can help you understand the nuance of any negative sentiment (such as anger, disappointment, or frustration) and explore why it might exist.
Access to deeper emotional insights like these is invaluable for building your brand for the better, both in the short term and for the long haul.
The most famous brands are the ones that have built emotional connections with their audiences. The right AI text mining tool can help you do just that.
What are the most common text mining applications for businesses?
While it may be one of the best-kept secrets for better business intelligence, word is getting out and we are seeing examples of automated text analyis being used across organizations to become more efficient in learning from qualitative text data.


For marketers, for example, text mining can offer a powerful way to understand target audiences, reflect on what customers love about their brand and offering, and analyze public opinion on their brand or competitors.
For product teams, quality NLP text mining can help identify any pain points customers have with their products or understand why people are choosing other products over theirs.
For people teams, text mining can help identify the real drivers of workforce satisfaction, by segmenting and analyzing employee survey responses.
And this is just scratching the surface of what’s possible. The power of AI text mining can be tailored to a host of business needs and objectives.
Embrace the data revolution now!
What types of text Mining tools are there?
There are two big-hitters when it comes to text analysis tools: bolt-on text analytics and standalone text analytics.
Bolt-on solutions largely offer data gathering and analysis for a particular type or source of text data. Think social listening tools like Brandwatch or survey platforms like Qualtrics, for example.
But there are two potential downfalls here.
Firstly, the only analysis a bolt-on solution can deliver is for a specific type of data (you can’t import customer reviews into a social listening tool). And secondly, text analytics is rarely the leading feature of these solutions, so outputs are going to be relatively unsophisticated (think word clouds and frequency tables that rarely offer new insights).
Fortunately, a high-quality standalone tool can work wonders.
AI text mining tools like Relative Insight enable users with a suite of text analysis and visualization capabilities that can be used to analyze text data from any source.
The team behind our platform are experts in natural language processing, computational linguistics, AI and more, and are laser-focused on generating the best possible insights from masses of text.
It’s this focus and expertise that enables a standalone tool like Relative Insight to get beneath the surface of your data to uncover the nuance in what people are really saying and feeling. This depth of analysis is essential to understanding and resonating with your target audiences.
The ability to seamlessly integrate with data providers and BI dashboard tools, make it easy to connect your specialized text mining solution with your existing research and insight text stack.
With all that said, what are you waiting for?